There’s a lot of chatter about big data and data gathering and analytics. Data quality is discussed less frequently, but that’s a mistake.
Problems with incoming information filter down through organizations to affect efficiency, collaboration, profitability, and customer satisfaction.
Business leaders have much to gain by investing in the means to collect and study information.
Quality must be prioritized just as highly as quantity, though. So, here are some of the essentials to maintaining data quality in a business.
1. Know How to Measure Data Quality
Data is considered high-quality when it serves the purpose for which it was created. How can businesses ensure that? Multiple stakeholders are often invested — clients, downstream workflows, and long-term planners.
To be truly useful, data must be:
- Accurate: Transcribed and transmitted without errors
- Complete: Not missing important values in data sets
- Consistent: Uniform in format and easily cross-referenced
- Relevant: Supports the process for which it was gathered
- Timely: Recent — or representative of the time at which it was captured
One study claims bad data costs companies around $100 for every inaccurate record. In three years, the price tag quickly reaches into the millions for a company with just 100,000 records.
2. Invest in Data Profiling
Sometimes the quality of a data set gets compromised right at the source. This isn’t always under your control — it could be information from business partners and third-party software. With industries like manufacturing and supply-chain management dealing with so many moving parts, a process to control and profile incoming data is a must-have.
Data-profiling tools remove the possibility of human errors and transcription problems by applying machine learning and automation. This helps maintain data quality by ensuring:
- Consistent data formatting
- Relevance to the question being asked or process being studied
- A lack of artefacts or abnormalities that could skew the results
3. Build a Pipeline That Weeds Out Duplicates
Multiple teams or individuals creating data using the same source and logic muddies the waters and makes compounding errors. Duplicates in data sets affect the ability to analyse the situation accurately and deliver value-adding results.
Eliminate this worry by clearly defining data pipelines, prioritizing communication, setting business rules for data creation, and creating easily understood, consistent processes for sharing data. Many companies invest in the following areas:
- A logically designed data pipeline set at the enterprise level and designed for consistent sharing and transparency across the organization.
- A detailed data-governance program with clear expectations and instructions for avoiding or eliminating duplicate information.
- A central data-management platform that ensures information can be audited routinely and helps avoid siloing within departments. Silos are a sure recipe for duplicate data sets.
Many companies need to consolidate their data-gathering processes and systems to maintain quality and eliminate processes resulting in duplicates.
4. Ensure Metadata Traceability
The U.S. Food and Drug Administration recognizes a lack of traceability as a top problem in data quality today. This is a problem even beyond the life-and-death stakes the FDA deals with daily, like approving new medications.
Sometimes problems happen in business that requires troubleshooting a data set and tracing the information to its source. Every company with a data pipeline must have the ability to trace lineage. Taking this step seriously means the time required to fix errors won’t increase as the company’s collection of records grows.
Making metadata traceability a part of creating your pipeline ensures downstream users of that information aren’t at the mercy of data scientists. They don’t have to trace things back through databases and separate departments or programs. Instead, individuals and teams can answer their own questions and do their own troubleshooting.
Metadata traceability involves different techniques depending on the organization and its needs. No matter the methodology, the result is the ability to understand the origin, journey, nature of, the relationship between, and the logic applied to the data coming down the pipeline. This might involve:
- Creating a unique key for each data set that can be carried downstream
- Using sequence numbers, like transaction IDs
- Deploying link tables describing relationships in the data set
- Pinning timestamps or version numbers to each data record
- Using a log table to record data changes
These steps involve using metadata identifiers as documentation not just of the provenance of the data, but the ways it’s been acted upon or transmitted.
5. Understand Data Requirements
This step involves the “why?” behind gathering data in the first place.
- What data does the department require? What value does it add?
- What specific questions does the client want to answer using data?
- What are you trying to troubleshoot or improve? How is it measured?
- What are the conditions under which you wish data to be collected?
Data isn’t valuable without defining these characteristics beforehand. Create formal documentation describing the nature and purpose of each information-gathering process. Ask department leads and clients: What do you want to find out or improve? What kinds of data, in which circumstances, will yield results?
Understanding the questions being asked by stakeholders, especially clients, is essential. Adopting data-analysis infrastructure without a shared and clearly understood goal is a recipe for mismanagement and wasted resources.
Helping companies understand data-gathering requirements and create expectations is a task often delegated to business analysts.
Maintain Data Quality Starting Today
Investing in maintaining data quality affects and improves the information’s entire life cycle. It becomes and stays more valuable over a longer period. From there, there’s no end to the ways data is useful in business today.
Author Profile
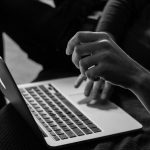
- Always on the chase after hot and trending London and UK business news that disrupt, inform and inspire.
Latest entries
Business Innovation NewsJune 27, 2025Fitness Education Online Nominated for Prestigious Business Awards UK Honour as Best Independent Education Service of 2025
Business Innovation NewsJune 13, 2025Tjurado Certified Translation Services in London Becomes A Trusted Partner For UK Authority Translations In Immigration, Education, Law And Medicine
EnvironmentJune 12, 2025From The Great Stink To Modern Dustmen – London’s Waste Management History investigated with Snappy Rubbish Removals
DictionaryMay 19, 2025Spring Clean – Meaning & Facts